The client – a leading oil company – wanted Zenitech’s help to create a predictive model that would tackle the problems it was having with human error in its PB gas tank sale management.
Accurate predictions are crucial for the business – especially regarding order quantity and timing.
The need to predict with confidence
The client faced several challenges:
- It had no refill capability - refilling gas tanks was outsourced to filling stations.
- There were limited PB gas tanks available, as fewer are being made.
- Filing stations also served competitors and could become so busy that orders were late, resulting in extra costs or missing fulfilment.
- Order numbers could fluctuate wildly. Field experts used their experience, Excel spreadsheets and the point-of-sale management system to estimate order numbers.
- Every retail order had a completion deadline of three to five days. Missing that deadline could lead to penalty payments and partnership churn, which impacts profitability.
The client needed to be able to predict demand accurately to ensure supply.
Zenitech’s approach
Zenitech’s project team—three data scientists and a project manager—worked closely with the client team to keep them fully updated on the project’s status, answer questions, and assimilate feedback.
During the project, we used PowerBI dashboards to visualise predictions and give a deeper understanding of the current state of the sales pipeline. The additional reports included different views – such as a sales territory-based view – and let the user filter the results. We also automated the data ingestion process and used machine learning to keep the dashboard’s underlying dataset fresh.
Excellent communication, a lack of siloes, and a highly-collaborative approach between Zenitech and the client were crucial to the project’s success.
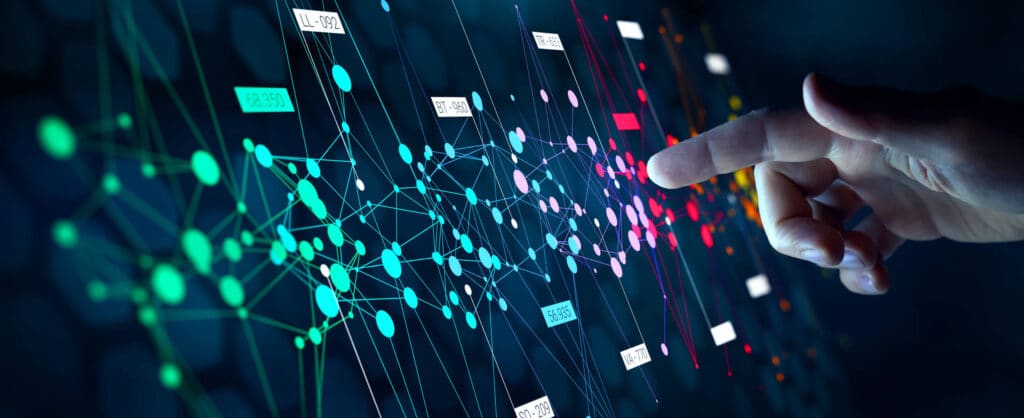
Getting the right data
The most important part of the development process was identifying the relevant data sources and data flows.
We started with sales data from SAP and one of the expert systems (Worx). The dataset included weather data and information about holidays – information that could impact delivery of orders.
After reviewing the data and the sales process, we worked closely with the client’s operational teams to gain a deeper understanding of business processes and available data.
This meant we could add data on orders, sales leads, sales deals, planning, pricing and depot information. The data was unstructured and in different formats (including customer- managed Excel tables and notes) and were not standardised between departments, so the team’s greatest challenge was cleaning and standardising the data to deliver accurate insights.
The result: accurate predictions based on good data
By the end of the project, the client had a machine learning model that achieved accurate results when predicting PB gas tank demand across a two-week period.
The new predictive modelling system resulted in more accurate sales predictions that decreased partnership churn significantly.
Subject area experts also gained a deeper understanding of the entire sales pipeline, improving communication and collaboration.
The project succeeded because of the effective communication between Zenitech and the client, the proactive attitude of the data science team, and the ability to adapt the project based on new data insights.